Benefits and Pitfalls of Data-Based Military Decisionmaking
Scott S. Haraburda
Sound and effective decisions, supported by reliable data, usually determines military operational success. Recent rapid advances in electronic instrumentation, equipment sensors, digital storage, and communication systems have generated large amounts of data. This deluge of digitized information provides military leaders innumerable data mining opportunities to extract hidden patterns in a wide diversity of situations.[1] From complex information contained in this varying data, visualization tools and other data science methods aid leaders, especially commanders and their staffs, in asking questions, developing solutions, and making decisions.
Recently, senior Army leaders demanded visualized access to massive amounts of data to enhance their decisionmaking, which quickly morphed into an ambition project called Army Leader Dashboard.[2] Nearly a thousand unique data sources from its initial efforts such as training databases, equipment inventories, and personnel records emerged. This proliferation of data appeared limitless and provided a staggering potential to enhance decisionmaking with valuable real-time information that crossed multiple functions throughout military organizations such as logistics, risks, and personnel. Notwithstanding, this soon revealed its data existed in silos, which were difficult to obtain and may not be reliable.
Perhaps, the inherent value behind data-driven decisions motivated China’s and Russia’s aggressive pursuit of seeking innovative ways to analyze data as evidenced in their significant investments in artificial intelligence (AI) for military purposes.[3] In response, the Department of Defense (DoD) created a strategy to incorporate AI into its own military decisionmaking processes. Besides developing a network of things, such as combat gear embedded with biometrics to help warfighters perform better, this strategy included updated organizational approaches, data standards, talent management, and operational processes. The ultimate goal was improvement of situational awareness and decisionmaking. Making this likewise a national endeavor, President Trump signed an executive order in early 2019 identifying AI as a priority for the United States (U.S.).[4]
Supporting this priority, the Army has established the Army AI Task Force within Army Futures Command with qualified professionals based at Carnegie Mellon University.[5] This task force will experiment, train, deploy, and test machine learning capabilities and workflows, all designed to improve readiness through data-driven military decisionmaking. The Army anticipates significant benefits arising from the extensive amount of resources the military will invest in data science tools. Yet, they might foolishly promote them as magical crystal balls capable of obtaining near-perfect intelligence. The real concern, though, is that military leaders may not comprehend significant risks associated with blindly using such tools.
Data-Based Decisions
Military operations involve multifaceted threats, many with differing agendas, reactive capabilities, and adaptive competencies in a non-static environment.[6] Further, uncertainty permeates operational plans, making it important that military leaders make timely and effective decisions based upon available information. To simplify analyses, leaders organize their data into political, military, economic, social, information, infrastructure, physical environment, and time operational variables. They also develop plans based upon mission, enemy, terrain and weather, troops and support available, time available, and civil considerations (METT-TC). Again adding to the complexity of military decisions, their plans address the nine traditional principles of war (objective, offensive, mass, economy of force, maneuver, unity of command, security, surprise, and simplicity) and three additional principles (restraint, perseverance and legitimacy).[7] Often neglected from these simple groups are socio-cultural intelligence aspects of the threat’s culture of beliefs, values, customs, and behaviors, all which impact military decisionmaking. Socio-economic issues, such as climate change, water / food shortages, and urbanization, also plague decisionmakers, especially when dealing with failed states and criminal syndicates.[8] Essentially, military decisions involve hundreds, if not thousands or even millions, of competing areas. The real challenge, then, is to discern the “vital few from the trivial many.”[9]
To formally plan and solve problems, military leaders use the military decisionmaking process, a seven-step process to understand situations, develop options, and reach decisions.[10] This process helps them think critically with available data, time, and resources. Its systematic attention to detail, which includes war gaming, sand tables, and computer simulations, is important since all planning is based upon imperfect knowledge and assumptions.[11] Improving mission success, military plans include outcome criteria such as measures of effectiveness and measures of performance. Since these plans involve people who often thwart order and efficiency, they should be both comprehensive and adaptable.[12]
Because of time constraints or inadequate personal technical skills, military leaders rely heavily upon their analysts to transform data into potential solutions to meet mission intent. Some analysts, though, are highly trained data science professionals capable of using sophisticated models in producing bewildering solutions to perplexing models. These models appear to be more mathematically elegant and impressive than effective simple solutions to actual problems.[13] However, since most leaders lack access to data scientists or other capable analysts, they require tools that are simple to use and provide credible results that can be trusted. No matter how compelling the analyses, though, recommended solutions are widely laden with judgmental biases, mental misperceptions and other dangerous risks.[14] Addressing these military risks, they use risk management (RM) tools by applying similar industrial tools used for safety risks, the significant difference being that military risks focus upon intended threats while safety risks focus upon unintended hazards.
Without the need for computational data assessments, the Army five-step RM process (identify hazards, assess hazards, develop controls / make risk decisions, implement controls, and supervise / evaluate) uses METT-TC and the Intelligence Preparation of the Battlefield to identify hazards. It also applies four categorical risk levels (extremely high, high, medium, low) to assess hazards.[15] This qualitative process further uses nine subjective assessments for severity (catastrophic, critical, moderate, negligible) and probability (frequent, likely, occasional, seldom, and unlikely), making it useful without needing sophisticated data science capabilities. This process is simpler than the DoD eight-stage RM process, which is similar but contains several additional documentation steps.[16] However, delaying a decision to obtain more information to make a better decision may actually increase risk, especially time-sensitive situations.[17] Hence, spending too much time on RM becomes counter-productive.
Of extreme benefit, analysts exploit tools to unlock secrets hidden in that information, available today measured and stored in digital bits. As a sophisticated integration of talent, tools, and techniques, data science is really an art of transforming data into actionable information needed for decisions.[18] To obtain data and report information, this science capitalizes upon data cleaning, data monitoring, reporting, and visualization processes.
Data scientists first conduct exploratory analyses to search data for trends, correlations, and relationships between measurements. Then, they use description analytics to understand operational aspects of the data, such as data summarization with basic statistics of mean and standard deviations to calculate a unit’s combat power. Using complex mathematical techniques together with machine learning and probability theory, they conduct predictive analytics to uncover relationships between data inputs and outcomes. Applying more complex techniques made up of modeling and simulation efforts, data scientists use prescriptive analytics to determine probabilities of potential outcomes based upon deliberate changes to inputs.[19] Despite sophisticated quantitative analyses using these different techniques, blindly trusting them without fully understanding its context, biases, and assumptions, can lead to perilous decisions.
If available, qualitative techniques, such as decision theory and war gaming, further improves data understanding. To reduce technical barriers and make it easier to provide data-relevant information for its users in geographically dispersed locations, computational support systems (data warehouses, data mining, virtual teams, knowledge management, and optimization software systems) augments these qualitative techniques.[20]
Benefits
The complex nature of our volatile modern world, with extreme quantities of data, burdens capabilities of traditional methods for military decisionmaking. As advancements in information technology (IT) increase, new opportunities emerge for data analytics. Historically, military leaders have relied upon simple data analysis, such as the number of enemy killed and the ratio of friendly to enemy troops, to make decisions. Today, they have access to instantaneous data not previously imagined, such as satellite-based tracking of troop locations and logistics pipeline statuses, all queried and analyzed by armies of analysts.[21]
Previously, data collection and analyses were expensive, requiring paper-based reports. With tremendous advancements in IT, including advancements in sensor and satellite technologies, military leaders began to obtain real-time access to data remotely on almost any topic.[22] This provided better opportunities in obtaining clearer pictures of situations that were swiftly adjusted to updated circumstances and uniquely tailored to specific missions. Advances in industrial data analytics likewise impacted military operations. For instance, in the mid-1990s, General Electric implemented a continuous improvement process, Six Sigma, as its primary management tool. Using updated IT networks with advanced number-crunching tools, this international company identified significant improvements that generated billions of dollars of shareholder value. Soon thereafter with desires to accomplish the same for its constrained resources, the DoD mandated Lean Six Sigma process throughout the military, impacting its decisionmaking capabilities for all of its activities.[23]
Case in point, environmental factors have a dramatic impact upon military actions, making it very important to possess accurate weather forecasts. Satellites, ground-based sensors, and weather spotters generate millions of environmental observations daily, running into petabytes (million gigabytes) of data each day. This includes continual measurements of “atmospheric pressure, wind speed/direction, temperature, dew point, relative humidity, liquid precipitation, freezing precipitation, cloud height/coverage, visibility, present weather, runway visual range, lightning detection, weather radars, soil moisture, river flow, coronal mass ejections, sea surface winds, electron density, cloud visible and infrared, and geomagnetic fluctuations, as well as other parameters.”[24] Though this may appear to create information overload, advanced analytical software and state-of-the-art computer systems have reduced weather analyses from several hours via hand to seconds via computer, providing decisionmakers near-real time information when and wherever needed. Data analytics enhance this capability by using more data points instantaneously to transform asymmetries of data into useful information.[25] Overcoming human limitations and biases, data analytics allow military leaders to make quicker decisions with more valid, dependable, and transparent information. Thus, data analytics provide valuable input into military decisions.[26]
Pitfalls
Although quantitative measures provide useful information, analysts often fail to select the vital metrics from the trivial many. Also, they may not even fully understand the metrics they selected. Measuring the percentage of large steel balls provides a striking analogy of this common problem. Given a quantity of one hundred steel balls, either large or small, three different analysts assessing the exact same set of balls can provide widely different values, ranging from 1 percent to 99 percent, which can drastically influence decisions. Concerned with quantity, 1 percent are large balls (one out of one hundred), which is an obvious and straightforward calculation. Still, there are numerous other methods to calculate percentages. For surface area, 50 percent are large balls (610 square inches cumulative for each). And for weight, 99 percent are large balls (405 pounds out of a total of 409 pounds). Even though percentages appear to be unitless, they assuredly have units such as by quantity, by surface area, and by weight in this analogy.
Even with fully defined metrics, the presentation of the data could mislead. Another analogy to illustrate this concern was calculation of baseball batting average (BA), which was a common metric to quantify batting performance. It was a simple analysis with BA defined as the fraction of hits within the number of times at bat (AB). In 2007, Boston Red Sox’s Jacoby Ellsbury displayed a BA of 0.353 (41 hits within 116 AB), compared to his teammate Mike Lowell who displayed a lower BA of 0.324 (191 hits within 589 AB). The following year, Ellsbury displayed a BA of 0.280 (155 hits within 554 AB) with Lowell displaying another lower BA of 0.274 (115 hits within 419 AB).[27] Despite Ellsbury having the higher BA each year and appearing counter-intuitive from the yearly analysis, Lowell was the better batter overall for that same two-year period. This is an example of the Reversal Paradox in which subsets of the data system can provide misleading information. In this baseball analogy, Ellsbury’s collective BA for both years was 0.293 (196 hits within 670 AB), while Lowell exhibited a higher BA at 0.304 (306 hits within 1008 BA).
People tend to seek information that supports what they already believe, and discount those that contradict their beliefs.[28] These are cognitive biases, such as groupthink and misconceptions, which often cause people to make judgment errors.[29] This impacts the approach in which military leaders receive information, whether it is the verbiage selected or the spatial, temporal, or categorical presentation manner. Aversion to risk is an example of cognitive bias, which might explain why a well-trained officer and veteran of the Mexican War, General George McClellan, with a larger and better equipped army was more concerned with avoiding failure than he was in winning battles during the 1862 Peninsula Campaign.[30] Another dangerous bias is jumping to an initial estimate and refusing to update it after receipt of contrary information. The Wehrmacht failed to update this for several years during World War II following a British deception plan that pretended a false division of twenty thousand troops garrisoned on the island of Cyprus, even in spite of their own contradictory analyses.[31]
Many people tend to refuse data with an inflexible certainty of belief, often invoking logical fallacies through arguments that contain chunks of accurate information taken out of context.[32] Others may be scientific illiterate and ‘cherry-pick’ data that supports their beliefs, ofttimes citing peer-reviewed articles as credible evidence proving their position. Serving as knowledgeable experts, some perform a relentless ‘paralysis of analysis’ with rigorous time-consuming studies to discourage dissenting opinions.
To fully understand the situation, military leaders had to maintain a good grasp of the analyses used, else they could become prone to making poor decisions as happened in 2013 when the International Security Assistance Force reported insurgent attacks had declined by 7 percent from using inaccurate information.[33] This inaccuracy stemmed from incorrect coding, which included data reporting bias, giving a false sense of reliability that led to unwarranted decisions. The following five historical military operations illuminated other dangers of making military decisions based solely upon quantifiable data.
Battle of Teutoburg
September of the year 9 CE in the Teutoburg Forest, German barbarians slaughtered three Roman legions. The deaths of its eighteen thousand legionaries were a result of the commander’s fatal decisions.[34] This catastrophic defeat stopped the expansion of the Roman Empire. Three years prior, Publis Quinctilius Varus became Governor of Germany where he failed to understand its German people and its lands. During his governorship, Varus attempted to Romanize them, and neglected to implement adequate force protection security measures.
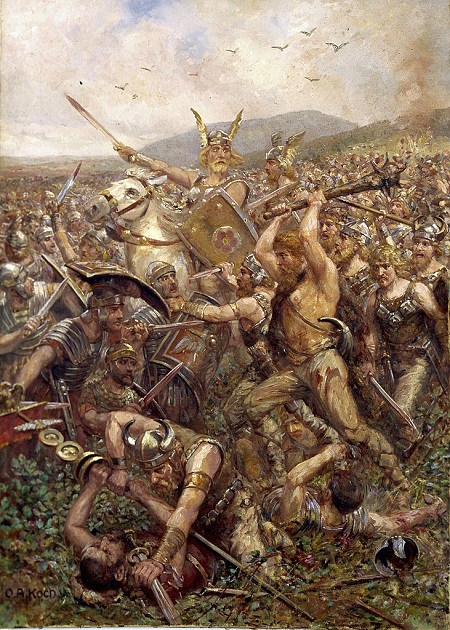
Figure 1. Germanic Warriors Storm the Field in the Varusschlacht (or Battle of Teutoburg Forest) in September 9 C.E. by Otto Albert Koch (1909)
By exploiting his ties to Rome and his knowledge of the Roman army, Arminius, a Cherusci chieftain and Roman auxiliary officer, capitalized upon Varus’s failures. He led a group of barbarians, inferior in discipline, weapons, and armor against the strongest military in the world. To accomplish this amazing feat, he aligned his tribe with three others, the Angrivarii, Bructeri, and Chatti, through their common subversive interests against their Roman subjugator. Despite evidence to the contrary when Segastes, a pro-Roman German, informed him of Arminius’s traitorous plans, Varus chose to disregard this vital information.
Instead, Varus viewed Arminius as a loyal servant of Rome who had received the rank of knight and Roman citizenship for his valor on the battlefield.[35] As his trusted advisor, Arminius convinced Varus to march his troops into hostile territory, a route that contained primitive trails that meandered through harsh terrain. This detour forced the march to become perilously extended, eventually growing to nearly eight miles long and highly vulnerable to attacks. After several days, the Roman legionaries entered a narrow passage between a hill and a huge swamp where the barbarians quickly attacked from behind trees and sand-mound barriers. Sensing no possibility for escape, Varus fell on his sword rather than face imminent torture as a prisoner. Most of his other commanders also committed suicide and abandoned their troops, who soon found themselves without leaders in the bloody ambush. Within a few hours, the ambushing barbarians destroyed more than ten percent of Rome’s invincible army, resulting in their hasty abandonment of its bases throughout Germany, clearly making this a strategic disaster.
Battle of Wabash River
Late into the eighteenth century, territorial settlers encroached upon Native American-occupied lands in the post-colonial Northwest Territory.[36] Infuriating them, they destroyed the native’s ancestral lands by chopping down trees and planting crops. In the fall of 1791 with fourteen hundred Soldiers from what remained of the disbanded Continental Army, Major General Arthur St. Clair, as Governor over those lands, launched a punitive expedition against the natives. On November 3, about one hundred miles directly north of now Cincinnati, his expedition reached the banks of the Wabash River, where they discovered indications of hostile natives nearby. Despite this dangerous data, St. Clair decided against implementing increased protective measures. The following morning with one thousand native warriors, Little Turtle attacked the similar-sized U.S. troops, many of whom quickly fled. Comprehending that artillery fire was their greatest threat, the natives prioritized their attacks against artillerymen. Once they neutralized the artillery threat, they attacked from all sides, giving U.S. Soldiers no effective way to overcome the relentless assault.
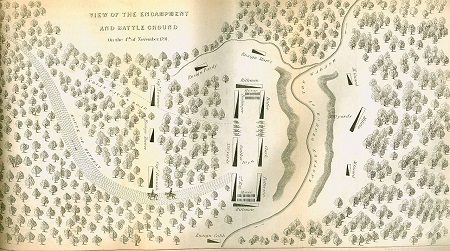
Figure 2. View of Major General St. Clair's Main Encampment by the Wabash River on November 4, 1791. Photo Credit: U.S. Army Military History Institute (2011)
Contributing to this slaughter were insufficient and shabby supplies, along with undependable officers who quarreled publicly and refused to communicate vital information.[37] Receiving meager intelligence, St. Clair did not even know the name of the river where the massacre of his troops occurred. In the end, Little Turtle’s shrewd leadership, native warrior competencies, and their resolve overcame the competence and courage of U.S. Soldiers. Still, as he underestimated his enemy, St. Clair made fatal decisions by not requiring increased security measures, not acting upon intelligence received, and not considering the actual capabilities of his own troops. As a result, the U.S. suffered a great loss of 657 dead and 271 wounded, losing nearly the entire unit. This which was a devastating defeat that resulted in more than double the losses that Lieutenant Colonel George A. Custer suffered along the Little Bighorn River almost a century later.[38]
Operation Market Garden
After nine days in September 1944, Operation Market Garden entered the history books as a colossal failure and delayed the end of the European Theater of World War II by months. Before the assault, Operation Overlord was complete, producing exhausted troops and depleted supplies, its transportation system unable to supply their future logistical demand.[39] On September 17, thousands of paratroopers landed behind enemy lines in the Netherlands to secure the Eindhoven-Arnhem corridor, making the intentions of Allies very clear. Although initially surprised from the airborne assault, German troops quickly regrouped, making it clear to the Allies on the ground that they were fighting highly motivated troops instead of disorganized ones assumed in the operational plans. Intelligence reports at the time had predicted the impending collapse of the Wehrmacht, but failed to predict their ability to quickly respond to military attacks.
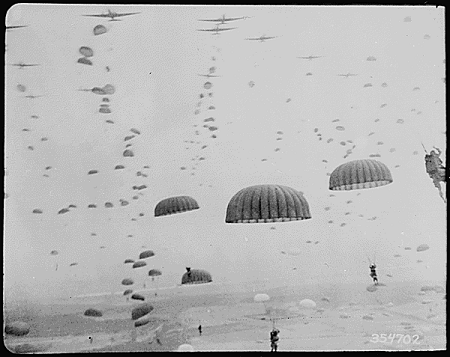
Figure 3. Photograph of Parachutes Opening Overhead as Waves of Paratroops Land in the Netherlands during Operations by 1st Allied Airborne Army in September 1944. Photo Credit: National Archives (September 1944)
The operation had two main objectives, to cross the Rhine and to neutralize the Ruhr Valley industries, along with securing a corridor for Allied ground attacks into Germany.[40] While planning for the operation, military leaders dismissed several warnings that their assumptions might be incorrect such as the Wehrmacht’s ability to resist an assault. Although the U.S. 82nd and the 101st Airborne divisions parachuted into their objectives successfully, the British 1st Airborne division jumped into a heavily fortified area, in the midst of two panzer divisions that were missing from the operational planning assumptions. Even though military leaders understood the intent of the operation, they had no method to measure success or failure during execution. Additionally, these plans identified no mission accomplishment indicators, and the tactical communication equipment operated poorly, making it extraordinarily difficult to communicate situational updates. For instance, many of the resupplies on the third day dropped into German hands without the logistics train knowing that the Allies did not control the area. After nine days and nearly twelve thousand casualties, the Allies withdrew.[41]
Vietnam War
From 1961 through 1968, Kennedy’s and Johnson’s escalation years during the Vietnam War, the Secretary of Defense (SecDef) implemented a nearly data-only decisionmaking process to manage military operations.[42] During World War II, Robert McNamara had served as an Army Air Forces lieutenant colonel where he exploited the rigor of statistical analysis to calculate efficiencies and effectiveness of its bombers. Following the war, he worked for Ford Motor Company, rising through the ranks to become its president in 1960, and then appointed SecDef a few months later.
Focused upon statistics to deduce truth from data, McNamara obsessed with narrow quantitative measures and neglected human interactions.[43] Throughout his career, he sought optimal methods to allocate resources in pursuit of mission objectives, a key objective for many data scientists and analysts today. Regrettably, his analysts found it difficult to quantify much of the Vietnam War data, such as motivation, hope, resentment, and other human emotions. Also, they could not verify available data for many of its analyses, much of it plagued with human biases. For example, many South Vietnamese military officers reported what they thought their U.S. counterparts wanted to hear, such as high body counts, instead of what had actually happened.
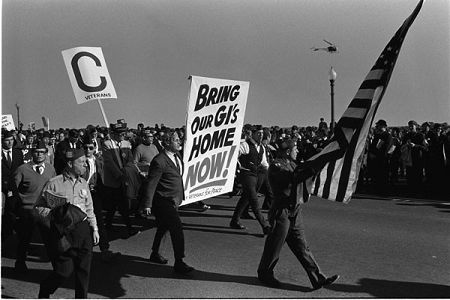
Figure 4. Photograph of Veterans for Peace at the March on the Pentagon on October 21, 1967. Photo Credit: Frank Wolfe, White House photographer.
The number of enemy killed, reported as body count, was McNamara’s measure to assess military progress. This was published daily in newspapers as public proof that U.S. Soldiers were winning the war. Mistrusting his experienced military officers while relying upon his civilian analysts, the ‘Whiz Kids’ from RAND corporation, a non-profit think tank, he felt he could comprehend what was happening in the field by staring at a bunch of numbers on spreadsheets and run the military like a civilian company.[44] Those analyses misled McNamara, who was infatuated with numerical data and its potential power to influence decisions. As the world discovered in the late 1960s, numbers alone were insufficient to gauge battlefield successes, especially when they were biased, misanalyzed, and misleading.
Fall of Mosul
In June 2014, fewer than one thousand Islamic State (IS) insurgents forced nearly sixty thousand Iraqi Army soldiers and federal police officers to flee Mosul, compelling roughly five hundred thousand civilians to abandon the city.[45] Before falling to IS, this city was a well-known commercial hub, straddling the Tigris River with upwards of two million residents.[46] The Iraqi Security Forces (ISF), with embedded U.S. military troops, had an effective unity of command with respected competent officers occupying key command billets. That was until 2011 with the U.S. military withdrawal from Mosul, along with the rest of Iraq, while they ignored dire warnings that it could cause the resurgence of IS insurgents.[47]
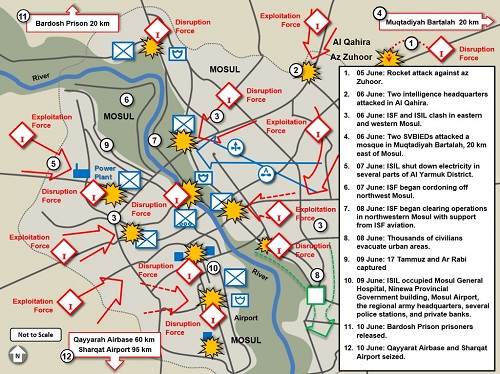
Figure 5. Dispersed Attack on the City of Mosul from June 6-10, 2014. Map obtained from U.S. Army TRADOC G-2 Unclassified Threat Tactics Report: Islamic State of Iraq and the Levant (February 2016).
From 2011 until 2014, ISF’s unity of command vanished, especially with the constant shuffling of commanders. This included four new commanders within two months of the attack, for the 2nd Iraqi Army Division, one of two divisions defending Mosul.[48] The selection board even removed one of its division commanders for failure to pay them a bribe. A key indication of the deteriorating situation in early 2014 was the number of monthly security incidents, which averaged around thirty per month prior to the U.S. military withdrawal and grew to more than three hundred each month. Command and control during the attack was even more disastrous, as ISF replaced the division commander twice during the week-long attack, providing virtually no command leadership over the frightened troops trying to defend Mosul.
Other difficulties that plagued ISF were officers who falsified personnel records by accounting for ‘ghost soldiers,’ those who paid them half their salaries in return for not reporting for duty. So, quantities of Iraqi soldiers available to defend the city were deeply exaggerated.[49] Even public information forewarning of the attack was either unnoticed or ignored. By 2014, the IS propaganda radically shifted from rival squabbles to topics addressing a global audience. This included the initiation of Islamic news reports in English and minute-long ‘mujatweet’ human interest stories in German.[50] Perhaps to alarm or threaten the residents of Mosul, IS issued the “Clanging of the Swords, Part Four” video a few weeks before they launched their attack. This video featured extreme footage of drive-by shootings, combat operations, explosions, and the fall of entire cities, which added to the escalating quantity of disastrous data ignored by ISF leaders.
Recommendations
These five historical military examples demonstrated that traditional quantitative metrics alone could lead to fatal decisions. In Teutoburg, the Roman military was the strongest in the world with superior discipline, weapons, and armor. Yet, the cunning and determination of a weaker force overwhelmed that dominance. On the banks of the Wabash River, untested assumptions and deliberate ignorance of critical data doomed a U.S. military commander facing an enemy with much better competencies and discipline.
During the largest airborne operation of World War II, the Allies soon discovered their failure to understand assumptions in their plans and to develop realistic contingencies doomed their attack. The Vietnam War confirmed that fighting a war with industrial-proven management techniques, such as statistical analysis, while ignoring human data from subject matter experts, could pummel military decisions into a strategic calamity. Finally, the undisciplined and unethical culture of troops protecting Mosul negated their overwhelming sixty to one numerical advantage and defied the three to one defense heuristic guideline, or “rule of thumb,” predicting their success.[51]
Before the onset of data analytics to uncover information, military leaders used heuristic guidelines to reduce complexity and to fill knowledge gaps, often based upon historical outcomes.[52] Additionally compounding decisionmaking today is the abundant quantity of data that masquerades as illusions of valuable information while more pertinent data remains misplaced and obscured.[53] Indeed, leaders should treat pertinent data that is both high-quality and reliable as strategic assets and force multipliers. Moreover, they should become open-minded and adopt adaptive leadership practices and methodologies to holistically address threats that rapidly evolve their tactics, techniques, and procedures.[54] Adaptive leaders should enjoy a mindset that commits to decisions, but do not become permanently linked to them.
Challenges facing military decisions clearly involve interdependences, uncertainties, and complexities such that military leaders need critical thinking to increase probability of desirable outcomes.[55] Since warfighting enterprise is a complex domain of human beings and their various emotional and cultural personalities, quantifying big data alone to make decisions is often inadequate.[56] Instead, effective analyses require qualitative information to uncover insights into the human domain of warfare. To address these challenges, military leaders should demand answers to the following questions from their analysts:
- Why were the selected metrics chosen instead of the many other metrics?
- What was the reliability of the data used?
- What human factors, such as culture and discipline, were used?
- What were the assumptions used, and how were they tested?
- What was the probability that the analysis was wrong?
- What were the risks if the analysis was wrong?
The military world is overflowing with data, which requires analyses brimming with subjective interpretations.[57] Because many analyses support pre-existing beliefs with cognitive biases, military leaders should designate competent data antagonists to uncover compelling evidence to challenge analyses. Finally, they should use analysts they trust or, better yet, become savvy with data science and other analytic tools themselves.
End Notes
[1] Damien Van Puyvelde, Stephen Coulthart, and M. Shahriar Hossain, “Beyond the buzzword: big data and national security decision-making,” International Affairs 93, no. 6 (2017): 1397-1416.
[2] Ellen Summey, “Creating Insight-Driven Decisions,” Army AL&T (Summer 2019): 14-20.
[3] Department of Defense. Summary of the 2018 Department of Defense Artificial Intelligence Strategy: Harnessing AI to Advance Our Security and Prosperity, February 2019. Retrieved https://media.defense.gov/2019/Feb/12/2002088963/-1/-1/1/SUMMARY-OF-DOD-AI-STRATEGY.PDF. “These investments threaten to erode our technological and operational advantages and destabilize the international order. As stewards of the security and prosperity of the U.S. public, the Department must leverage the creativity and agility of the United States to address the technical, ethical, and societal challenges posed by AI and realize its opportunities to preserve the peace and security for future generations.”
[4] “Executive Order 13859 of February 11, 2019, Maintaining American Leadership in Artificial Intelligence,” 84 Federal Register 3967.
[5] Army Directive 2018-18, “Army Artificial Intelligence Task Force in Support of the Department of Defense Joint Artificial Intelligence Center,” October 2, 2018.
[6] Department of the Army Doctrine Reference Publication (ADRP) 5-0. The Operations Process (May 2012): 1-1 to 1-9.
[7] Department of the Defense Joint Publication 3-0. Joint Operations. w/change 1 (22 October 2018): I-2.
[8] Robert R. Tomes, “Socio-Cultural Intelligence and National Security,” Parameters 45, no. 2 (2015): 61-76.
[9] Joseph M. Juran, Juran on Leadership for Quality: An Executive Handbook, (New York, NY: The Free Press, 1989): 136. Juran and Vilfredo Pareto identified the principle of the “vital few and trivial many,” leading to the term “Pareto principle,” which states that, for many events, roughly 80% of the effects come from 20% of the causes.
[10] Department of the Army (DA) Field Manual (FM) 6-0. Commander and Staff Organization and Operations. chg. 2 (April 2016): 9-1, 9-4.
[11] Center for Army Lessons Learned (CALL) Report 15-06. Military Decisionmaking Process: Lessons and Best Practices (March 2015): 1, 25, 41, and 66.
[12] Department of the Army (DA) Army Doctrine Reference Publication (ADRP) 6-0. Mission Command (May 2012): 1-2, 2-5, and 2-8.
[13] Terry Williams, “The Contribution of Mathematical Modelling to the Practice of Project Management,” IMA Journal of Management Mathematics 14, no. 1 (2003): 3–30.
[14] Edouard Kujawski and Gregory A. Miller, “Quantitative Risk-Based Analysis for Military Counterterrorism Systems,” Systems Engineering 10, no. 4 (2007): 273-289.
[15] Army Techniques Publication (ATP) 5-19, Risk Management, chg. 1 (April 2014): 1-3 to 1-10.
[16] US Department of Defense, Standard Practice, System Safety: Environment, Safety, and Occupational Health, Risk Management Methodology for Systems Engineering, MIL-STD-882E (11 May 2012): 9-17.
[17] Matthew R. Myer and Jason R. Lojka, “On Risk: Risk and Decision Making in Military Combat and Training Environments,” (Master’s thesis, Naval Postgraduate School, 2012): 63.
[18] Booz Allen Hamilton, The Field Guide to Data Science, 2nd ed. (McLean, VA, 2015): 4, 21, 28, 51, and 56.
[19] National Academies of Sciences, Engineering, and Medicine, Strengthening Data Science Methods for Department of Defense Personnel and Readiness Missions. (Washington, DC: The National Academies Press, 2017): 53, 62-65
[20] J.P. Shim, Merrill Warkentin, James F. Courtney, Daniel J. Power, Ramesh Sharda, and Christer Carlsson, “Past, present, and future of decision support technology,” Decision Support Systems 33, no. 2 (2002): 111-126.
[21] Mark Van Horn, “Big Data War Games Necessary for Winning Future Wars,” Military Review Exclusive Online (September 2016), https://www.armyupress.army.mil/Journals/Military-Review/Online-Exclusive/2016-Online-Exclusive-Articles/Big-Data-War-Games
[22] Daniel C. Esty and Reece Rushing, “The Promise of Data-Driven Policymaking in the Information Age,” Center for American Progress (April 2007): 2, 4, 6, and 10. https://www.americanprogress.org/wp-content/uploads/issues/2007/04/pdf/data_driven_policy_report.pdf
[23] Department of Defense Instruction 5010.43, “Implementation and Management of the DoD-Wide Continuous Process Improvement/Lean Six Sigma (CPI/LSS) Program,” (17 July 2009).
[24] Ralph O. Stoffler, “The Evolution of Environmental Data Analytics in Military Operations,” Chapter 7 in Military Applications of Data Analytics, ed. Kevin Huggins (Boca Raton, FL: CRC Press, 2019): 113-128.
[25] Nicolaus Henke, Jacques Bughin, Michael Chui, James Manyika, Tamim Saleh, Bill Wiseman, and Guru Sethupathy, “The Age of Analytics: Competing in a Data-Driven World,” McKinsey Global Institute (December 2016): 10, 11, and 75.
[26] Edward M. Masha, “The Case for Data Driven Strategic Decision Making,” European Journal of Business and Management 6, no. 29 (2014): 137-146.
[27] Data retrieved from Baseball Reference website: https://www.baseball-reference.com/
[28] C.W. Von Bergen and Martin S. Bressler, “Confirmation Bias in Entrepreneurship,” Journal of Management Policy and Practice 19, no. 3 (2018): 74-84.
[29] David Arnott, “Cognitive Biases and Decision Support Systems Development: A Design Science Approach,” Information Systems Journal 16, no. 1 (2006): 55-78.
[30] Michael J. Janser, “Cognitive Biases in Military Decision Making,” Civilian Fellowship Research Paper, U.S. Army War College (14 June 2007): 9-10. https://apps.dtic.mil/dtic/tr/fulltext/u2/a493560.pdf
[31] Blair S. Williams, “Heuristics and Biases in Military Decision Making,” Military Review 90, no. 5 (2010): 40-52.
[32] Greggory J. Favre, “The ethical imperative of reason: how anti-intellectualism, denialism, and apathy threaten national security” (Master’s Thesis, Naval Postgraduate School, 2016): 8, 9, 10, 49, and 53.
[33] Hans Liwång, Marika Ericson, and Martin Bang, “An Examination of the Implementation of Risk Based Approaches in Military Operations,” Journal of Military Studies 5, no 2 (2014): 38-64.
[34] James L. Venckus, “Rome in the Teutoburg Forest” (Master’s Thesis, U.S. Army Command and General Staff College, 2009): 1, 2, and 38-43.
[35] Fergus M. Bordewich, “The Ambush That Changed History,” Smithsonian Magazine 37, no. 6 (2005): 74-81.
[36] James T. Currie, “The First Congressional Investigation: St. Clair's Military Disaster of 1791,” Parameters 20, no. 4 (1990): 95-102.
[37] Leroy V. Eid, “American Indian Military Leadership: St. Clair's 1791 Defeat,” The Journal of Military History 57, no. 1 (1993): 71-88.
[38] U.S. Army Center of Military History, “Indian War Campaigns,” website https://history.army.mil/html/reference/army_flag/iw.html “The 7th Cavalry's total losses in this action (including Custer's detachment) were: 12 officers, 247 enlisted men, 5 civilians, and 3 Indian scouts killed; 2 officers and 51 enlisted men wounded.”
[39] Joel J. Jeffson, “Operation Market-Garden: Ultra Intelligence Ignored,” (Master’s thesis, U.S. Army Command and General Staff College, 2002): 5, 11, 12, 65-66.
[40] Carl H. Builder, Steven C. Bankes, Richard Nordin, Command Concepts: A Theory Derived from the Practice of Command and Control, (Santa Monica, CA: RAND, 1999): 104-116.
[41] Charles B. MacDonald, The Siegfried Line Campaign, (Washington, DC: Office of the Chief of Military History, Department of the Army, 1993): 199.
[42] Graham A. Cosmas, The Joint Chiefs of Staff and The War in Vietnam: 1960–1968: Part 2, (Washington, DC: Office of Joint History Office of the Chairman of the Joint Chiefs of Staff, 2012): 2.
[43] Phil Rosenzweig, “Robert S. McNamara and the Evolution of Modern Management,” Harvard Business Review 88, no. 12 (2010): 87-93.
[44] Kenneth Cukier and Viktor Mayer-Schönberger, “The Dictatorship of Data,” MIT Technology Review (31 May 2013). https://www.technologyreview.com/s/514591/the-dictatorship-of-data/
[45] Rod Nordland, “Iraqi Forces Attack Mosul, a Beleaguered Stronghold for ISIS,” The New York Times (16 October 2016) https://www.nytimes.com/2016/10/17/world/middleeast/in-isis-held-mosul-beheadings-and-hints-of-resistance-as-battle-nears.html; Tim Arango, “Iraqis Who Fled Mosul Say They Prefer Militants to Government,” The New York Times (12 October 2014) https://www.nytimes.com/2014/06/13/world/middleeast/iraqis-fled-mosul-for-home-after-militant-group-swarmed-the-city.html
[46] Shelly Culbertson and Linda Robinson, Making Victory Count After Defeating ISIS: Stabilization Challenges in Mosul and Beyond (Santa Monica, CA: RAND Corp., 2017): 4, 65.
[47] Tim Arango and Michael S. Schmidt, “Last Convoy of American Troops Leaves Iraq,” The New York Times (18 December 2011) https://www.nytimes.com/2011/12/19/world/middleeast/last-convoy-of-american-troops-leaves-iraq.html
[48] Michael Knights, “How to Secure Mosul: Lessons from 2008-2014,” Research Notes no. 38, The Washington Institute for Near East Policy (October 2016). Retrieved https://www.washingtoninstitute.org/uploads/Documents/pubs/ResearchNote38-Knights.pdf
[49] Ned Parker, Isabel Coles, and Raheem Salman, “Inside the Fall of Mosul,” Pulitzer Prize Entry for International Reporting (Baghdad, October 14, 2014) https://www.pulitzer.org/files/2015/international-reporting/reutersisis/01reutersisis2015.pdf
[50] Alberto M. Fernandez, “Here to stay and growing: Combating ISIS propaganda networks,” The Brookings Project on U.S. Relations with the Islamic World U.S.-Islamic World Forum Papers 2015 (Washington, DC: Brookings Institute, October 2015): 8.
[51] T.N. Dupuy, “Combat Data and the 3:1 Rule,” International Security 14, no. 1 (Summer, 1989): 195-201. “The 3:1 rule is a crude rule of thumb that suggests that an attacker is likely to be successful if he has an overall numerical strength superiority of three to one over the defender.”
[52] Jenny Stacy, “Ask the Right Questions,” Army AL&T (Summer 2019): 85-88.
[53] Daniel E. Stimpson, “So Much Data, So Little Time,” Army AL&T (Summer 2019): 122-127.
[54] William J. Cojocar, “Adaptive Leadership in the Military Decision Making Process,” Military Review 91, no. 6 (2011): 29-34.
[55] Richard Runyon, Fred Tan Wel Shi, and Jivarani Govindarajoo, “Developing Adaptive Leaders Through Critical Thinking” in Adaptive Leadership in The Military Context: International Perspectives, eds. Douglas Lindsay and Dave Woycheshin (Kingston, Ontario: Canadian Defence Academy Press, 2014): 81-100.
[56] Aleks Nesic and Arnel P. David, “Operationalizing the Science of the Human Domain in Great Power Competition for Special Operations Forces,” Small Wars Journal 15, no. 4 (2019). https://smallwarsjournal.com/jrnl/art/operationalizing-science-human-domain-great-power-competition-special-operations-forces
[57] Sam Ransbotham, “Better Decision Making with Objective Data is Impossible,” MIT Sloan Management Review (28 July 2015). https://sloanreview.mit.edu/article/for-better-decision-making-look-at-facts-not-data/
About the Author(s)
Comments
The customer support team of…
The customer support team of term paper writing service is dedicatedly working to resolving the problems of the students worldwide. We know how tough writing an assignment can be. Maybe you're trying to break into a new grade boundary; maybe you can't figure out how to structure your work; or maybe it's just that you have to talk about a topic that you're really struggling to understand!
We are committed to…
We are committed to providing our clients with exceptional solutions while offering web design and development services, graphic design services, organic SEO services, social media services, digital marketing services, server management services and Graphic Design Company in USA.